The advent of highly advanced hash technologies known as local descriptor hashes have started a revolution in the way hotline analysts and content moderators (hereafter referred to as analysts) review reported child sexual abuse material (CSAM). By extracting visual fingerprints from known images and videos, analysts are now able to automatically identify CSAM images and videos that have been dramatically altered to avoid detection, effectively streamlining what has until recently been an arduous and often traumatizing process.
In a nutshell, hash technologies that use local descriptors make it possible for analysts to spend more time reviewing new potential CSAM cases, and much less time checking whether the reported content is known and therefore already reported to law enforcement.
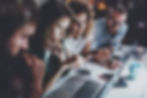
Included in this article:
Videntifier’s approach to hash matching with local descriptor hashes
Hash matching is the process of comparing one image’s visual fingerprint(s) with another to determine the level of similarity between the two, and is the primary method analysts rely on when using hash technologies to sift through reported CSAM. Hash matching is often used to identify newly reported CSAM against known harmful images indexed in a database, helping analysts to quickly sort through previously reported images and allocate resources to unknown cases. And, depending on the sophistication and power of the hash type being used, analysts can even use hash matching to accurately identify altered images (blurred, cropped, rotated, grayscale, etc.) against their indexed originals, enabling analysts to quickly distinguish whether the original of a newly reported altered image has already been reported to the authorities and lighten the workload for hotlines, law enforcement, and industry platforms.
However, as suggested above, not all hashes are created equal, and tend to vary in their ability to accurately match visual content. While less advanced hash types such as strict hashes or perceptual hashes are able to aid analysts in rudimentary hash matching, they are not quite able to achieve the level of accuracy and robustness offered by local descriptor hashes. Videntifier’s technology utilizes local descriptor hashes to provide a comprehensive solution for effective hash matching, designed to execute fast and accurate identification of video content in particular.
Let’s take a look at how exactly our approach to hash matching with local descriptors works.
How Videntifier uses local descriptors to improve hash matching
Videntifier's local descriptor technology identifies hundreds of visual interest points per each image or video frame to accurately match visual content against known originals. Each interest point is encoded into a series of numbers that describe the visual features around that point. The visual fingerprint for that image/video frame is then formed by gathering all the encoded interest points. If two images/frames are visually similar, then the comparison of their visual fingerprints will yield a high visual similarity signal. Because of the high granularity of Videntifier's hashing technology, the identification system offers unrivaled accuracy and robustness when it comes to identifying heavily altered content.
The picture below shows the visual fingerprints (with their location represented by yellow dots) of two similar images/frames and how they match (red lines).

Because of how thoroughly our local descriptors can fingerprint visual content, analysts are able to accurately match altered visual content against known originals. Let’s take a look at the different ways analysts can benefit from hash matching with local descriptors.
Benefits of hash matching with Videntifier’s local descriptors
Partial image matching
Let’s say an analyst is reviewing this piece of newly reported
content:
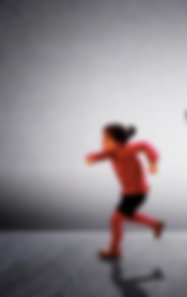
Initially, this particular image might appear completely harmless—just an innocent picture of a little girl running. If the analyst is relying on manual processes to determine whether the subject in the image is being harmed, it would likely be given the green light and labeled innocuous.
But when the analyst searches the image’s hash code against her known hash database, an entirely different context appears:
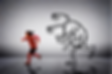
The newly reported image’s visual fingerprints register as similar to that of the database’s indexed image above, indicating that the image that was previously thought to be harmless is actually cropped to avoid detection. By using our technology to measure the newly reported image’s numerical value against the indexed image living in the known database, the analyst is able to recover the missing context and quickly label the newly reported image as known harmful content and move forward with her reviews.

Similarity clustering
With the detailed matching information made possible by Videntifier’s local descriptor hashes, an analyst can automatically order matches by match strength. This opens the potential for manual deduplication, where a visually modified image can be grouped with the original image. Using local descriptor hashes in this way is known as similarity clustering.

Similarity clustering allows matching of related images, either against significantly modified images–for example, cropped or rotated images–or against images taken in the same location. Being able to match related images can reveal connections between CSAM cases previously thought to be completely separate, providing analysts with a streamlined tool for helping law enforcement improve the efficiency of investigations.
Hash matching videos
Identifying content with Videntifier’s local descriptor hash technology works the same way for videos as it does for images; a visual fingerprint is extracted from each of the reported video’s frames, which can then be measured against the numerical values extracted from frames of known videos. This goes for altered content as well, including rotation, mirroring, cropping, and all other types mentioned above, as demonstrated in the video below.
Video processing and preparing for review
When processing videos, Videntifier groups and stores visual information as scenes, instead of storing information about each individual frame. This allows Videntifier to store detailed match information about the content while avoiding storing redundant information. The match information includes timestamps, so when matching content, Videntifier can provide details down to a second on exactly where in the content a match is taking place. This allows Videntifier technology to match short clips of videos, even single frames, to the original videos.

Exposure reduction
As illustrated above, using Videntifier’s local descriptor technology to hash match puts a wide variety of match types at an analyst’s disposal, dramatically decreasing their daily workload and improving the efficiency of the review process. But what comes part and parcel with this drastic cut in workload is a significant reduction in the amount of CSAM an analyst is exposed to everyday. Which, after years of being exposed, can have a considerably negative effect on the analyst’s psyche.
So, for example, let’s say a trailer-style video exists in the analyst’s hotline database, but a longer version of that same video has recently been reported. In such a case, all of the previously identified content can be blurred so the analyst only has to look at the new, unknown part of the video, saving the analyst from having to repeatedly expose themself to the known content as they review the new content.

Providing analysts with the ideal approach
To summarize, Videntifier’s approach to local descriptor hash technology works to dramatically improve hash matching. With thorough extractions of visual interest points from images and videos, optimized hash matching can streamline analysts’ review process by offering:
Hash match images: Identify the full context of harmful content previously thought innocent.
Similarity clustering: Identify images taken in the same location.
Video match types: Accurately search reported videos against your own database or databases of known content, such as the NCMEC database, through Videntifier technology.
Reduce exposure: Save analysts from repeated exposure to traumatizing videos by blurring the clips that have already been reviewed.